Harnessing Machine Learning Spam Detection for Business Success
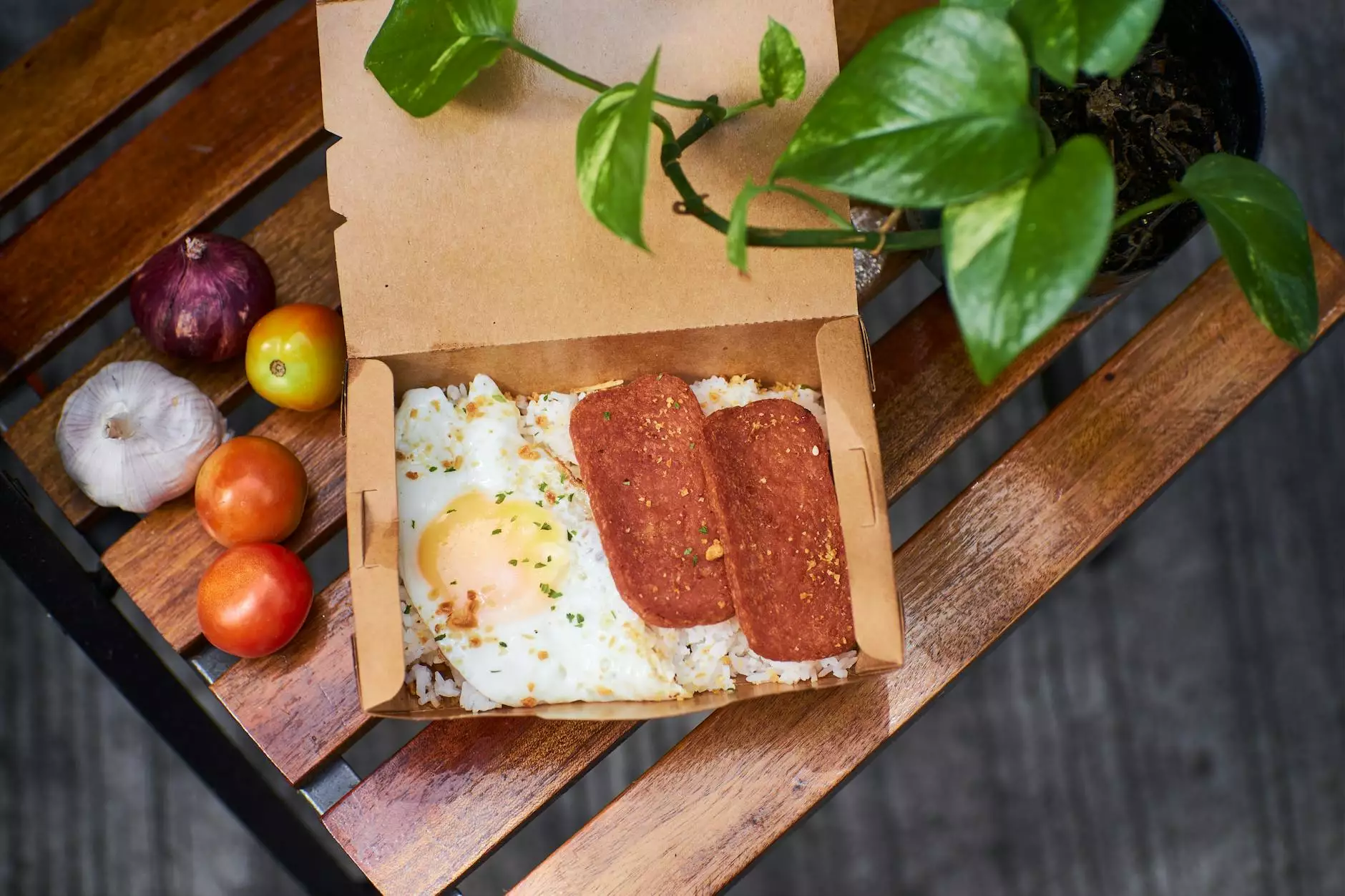
In today's digital landscape, businesses are inundated with information. While this presents immense opportunities, it also comes with challenges, particularly in the form of spam. As companies like Spambrella focus on IT Services & Computer Repair and Security Systems, implementing effective spam detection is crucial.
Understanding the Challenge of Spam
Spam is a pervasive issue that affects all forms of digital communications, including email, social media, and websites. It can take many forms, from unsolicited emails to deceptive advertisements. The consequences of spam are significant:
- Reduced productivity: Employees wasting time filtering through spam.
- Security risks: Spam can contain malicious links or attachments.
- Brand reputation damage: Spam can erode consumer trust in an organization.
The Role of Machine Learning in Spam Detection
This is where machine learning comes into play. By leveraging algorithms and statistical models, machine learning can significantly enhance the ability to detect and filter out spam. Here are some key benefits:
1. Improved Accuracy
Traditional spam filters often rely on a set of predefined rules and criteria. However, machine learning spam detection systems can learn from patterns in data, leading to:
- Adaptive filtering: Systems can evolve based on new spam tactics.
- Reduced false positives: Better accuracy means legitimate emails are less likely to be marked as spam.
2. Real-time Analysis
Machine learning systems can analyze incoming data in real-time. This allows for:
- Faster threat detection: Identifying spam before it reaches the inbox.
- Dynamic response capabilities: Adapting filtering techniques on-the-fly as new spam emerges.
3. Enhanced Security
Incorporating machine learning in systems can result in a more secure IT infrastructure. This includes:
- Threat intelligence: Collaborating with other machine learning systems to share data on emerging threats.
- Advanced phishing detection: Identifying deceptive emails that mimic legitimate sources.
Implementation Strategies for Machine Learning Spam Detection
To successfully incorporate machine learning spam detection into your operations, consider the following strategies:
1. Data Collection and Preparation
Gathering a comprehensive dataset is foundational. This includes:
- Historical spam data: Analyze previous spam messages.
- Legitimate communications: Collect samples of harmless emails for comparison.
2. Choosing the Right Algorithms
Several algorithms are prevalent in spam detection, including:
- Naive Bayes: A probabilistic classifier effective in text classification.
- Support Vector Machines: Useful for large feature spaces.
- Neural Networks: Capable of capturing complex patterns and nonlinear relationships.
3. Continuous Learning and Improvement
The digital environment is continuously evolving. Machine learning systems should:
- Regularly update their models: Incorporate new data frequently.
- Employ A/B testing: Experiment with different filtering techniques for effectiveness.
Success Stories: Companies Using Machine Learning for Spam Detection
Numerous organizations have successfully implemented machine learning spam detection, leading to improved operational efficiency:
Case Study: Google
Google's Gmail employs advanced machine learning algorithms to filter spam, achieving impressive accuracy and user satisfaction. By continuously learning from user interactions, it refines its spam detection capabilities over time.
Case Study: Microsoft
Microsoft integrates machine learning in its Office 365 platform, significantly reducing spam and phishing attempts. Their system utilizes a blend of algorithms that adapt based on user feedback.
The Future of Machine Learning Spam Detection
As we look ahead, the importance of machine learning in combating spam will only grow. Here are some anticipated trends:
- Increased use of AI: Artificial intelligence will play a larger role in understanding and predicting spam trends.
- Greater emphasis on privacy: Systems must balance effective spam filtering while adhering to privacy regulations.
- Integration with other security measures: Machine learning spam detection will become part of a broader cybersecurity strategy.
Conclusion
In conclusion, machine learning spam detection represents a critical advancement in the fight against digital clutter and security threats. By harnessing this technology, businesses can not only protect their operations but also enhance their overall productivity and user experience. Companies like Spambrella are leading the charge in offering robust solutions that combine IT services and security systems to keep your business safe from the ever-evolving spam landscape.
Investing in machine learning spam detection is not just an option; it's becoming a necessity for forward-thinking organizations that wish to remain competitive in a rapidly digitalizing world. Embrace this technology today to safeguard your business tomorrow.